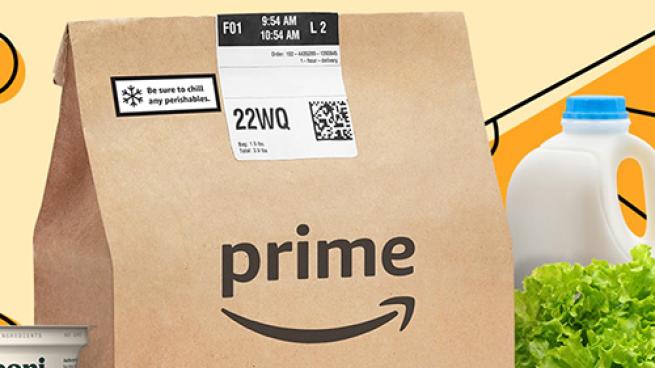
Amazon is ensuring Amazon Fresh brick-and-mortar stores and online deliveries both meet localized customer demand.
Amazon Fresh, a high-tech grocery concept that will soon encompass seven physical stores and also provides fresh product in as little as one to two hours in many parts of the country, offers tens of thousands of products. The Amazon Fresh assortment includes a complete grocery selection, as well as everyday essentials, gifts, and other items.
As detailed in a recent post on the Amazon Science blog, Amazon is developing machine learning models to enhance the Amazon Fresh shopping experience across channels. As part of this mandate, the Amazon Fresh team develops forecasting models, which are critical for grocery products with a limited shelf life.
Amazon Fresh’s suppliers include manufacturers, distributors, and local farmers. To maintain high in-stock selection while also ensuring minimum waste, the retailer must ensure that it purchases products from suppliers with the most relevant selection and optimal delivery times. Scientists at Amazon have developed time series models to enable the Amazon Fresh vendor-selection system to arrive at purchasing decisions in an automated way. The algorithm seeks to continually help Amazon Fresh customers gain access to the highest-quality products at the lowest possible price.
The Amazon Fresh forecasting models try to determine what the demand for a particular product will be in a particular marketplace at a certain moment in time. The models allow the company to determine which vendor to buy a product from while taking factors like quality, shipping times, and price into account. The Amazon Elastic Map Reduce (EMR) cloud-based service processes large amounts of data from multiple inputs on the supply and demand side.
Amazon EMR offers built-in machine learning tools, including Apache Spark MLlib, TensorFlow, and Apache MXNet, to create and customize predictive analytics toolsets. In-house-developed forecasting models have helped Amazon Fresh improve inventory availability by 15% after the outbreak of COVID-19.
To deal with the high likelihood that grocery shoppers will seek product substitutions when a first choice is out of stock, a machine learning model generates pairings between different products. The model assigns a rank to each pairing, using human opinion as a source of training data. This approach allows Amazon Fresh to analyze the rankings between different pairings, and recommend the most apt substitutions.
Machine learning also helps Amazon Fresh automatically detect mismatches between key product attributes and correcting them. In addition to uncovering pricing anomalies, machine learning models can help differentiate between a can of soda as compared to a six pack for the same brand, and also identify mismatches between product images and titles.
“Just like any other team at Amazon, we are taking a customer-centric approach to this challenge,” said Wei Gao, Amazon VP grocery, product, and supply chain. “We want to anticipate our customers’ needs, so that grocery shopping is simple and convenient. The work of our scientists is helping us achieve that mission."
January 13, 2021 at 02:13AM
https://chainstoreage.com/amazon-applies-machine-learning-fresh-grocery-experience
Amazon applies machine learning to fresh grocery experience - Chain Store Age
https://news.google.com/search?q=fresh&hl=en-US&gl=US&ceid=US:en
No comments:
Post a Comment